Ep 1: The Future of AI in Banking Risk and Compliance
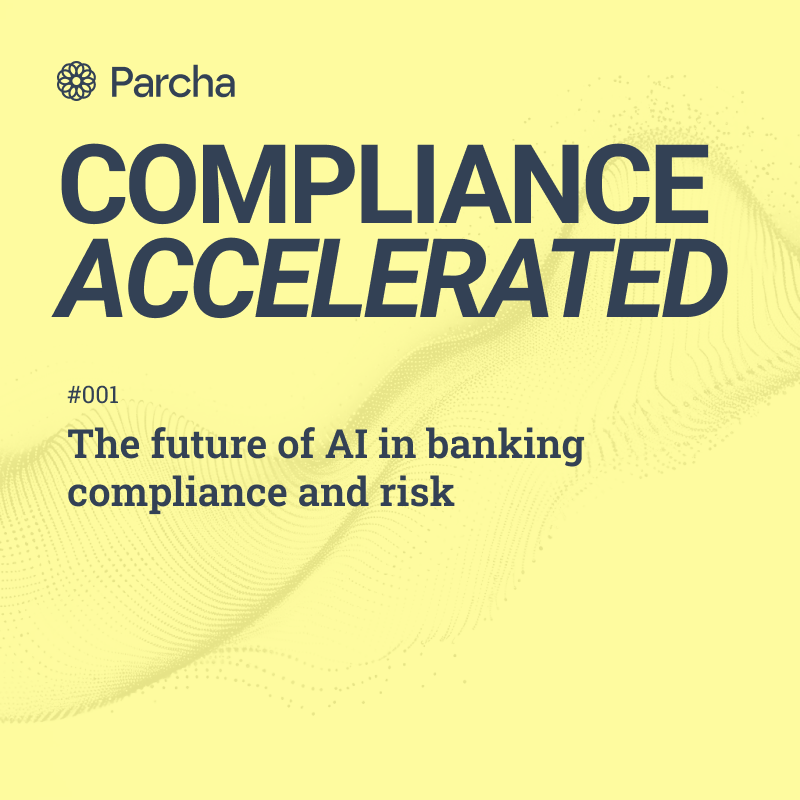
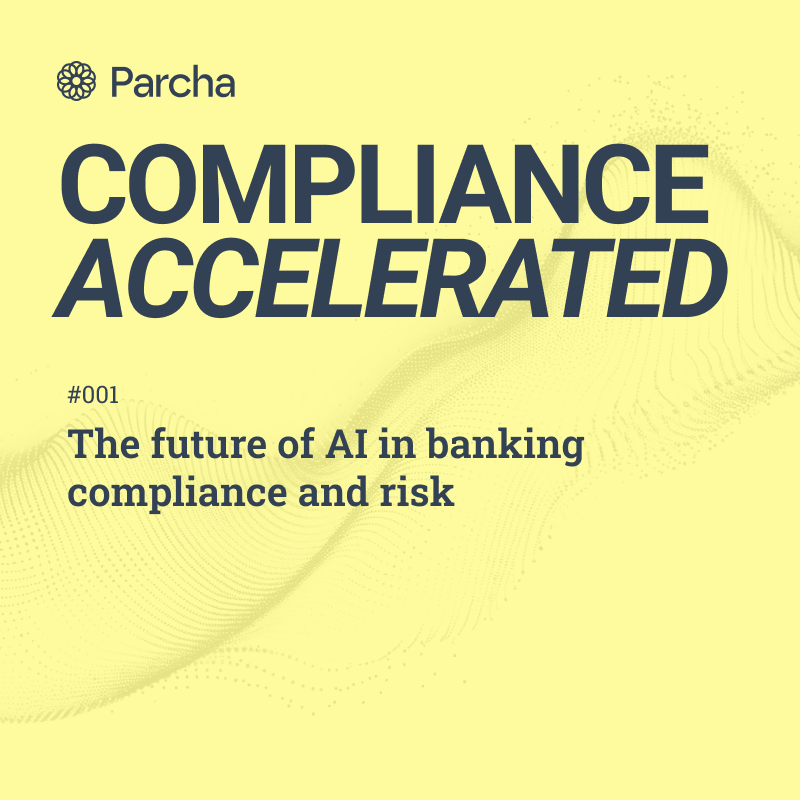
Compliance Accelerated is a podcast about the future of compliance and risk in banking and fintech. It is completely generated by AI using Google's NotebookLM and edited by our team at Parcha.
In this episode, we explore how generative AI is set to transform risk management within the banking sector, focusing on insights from McKinsey's March 2024 report. We discuss practical applications such as virtual experts, manual process automation, and six key impact areas: regulatory compliance, financial crime, credit risk, modeling and data analytics, cyber risk, and climate risk. We also delve into the potential risks associated with this technology, including impaired fairness, intellectual property issues, privacy concerns, and technical reliability. Finally, we cover McKinsey's seven-point plan for banks to strategically and responsibly integrate generative AI into their operations.
Here's the report if you want to read it yourself:
Timestamps
00:00 Introduction: The Overwhelming World of Banking Regulations
00:16 Generative AI: A Game Changer for Banks
01:16 Practical Applications of Generative AI
02:31 Key Areas of Impact in Banking
06:54 Potential Risks and Challenges of Generative AI
09:54 Strategic Approaches for Banks
11:27 Conclusion: The Future of AI in Banking
Transcript
Alice: Okay, so picture this, you're a bank manager, and you're drowning in regulations just trying to keep up with every possible financial threat out there.
Bob: Yeah, it's a lot to handle. Especially with how fast things change in finance these days.
Alice: And that is exactly what today's deep dive is all about, generative AI. We're talking about the type of AI that doesn't just, you know, crunch numbers, it actually learns, adapts, and can even make decisions. We're diving deep into a McKinsey report from March 2024 called Generative AI for Banks, and they are predicting a total revolution in how banks manage risk.
Bob: Yeah, they think this is going to be huge.
Alice: They really don't hold back in this report. McKinsey is saying, This tech could completely change how banks manage risk and in the next three to five years, which is a really short timeline when you consider how massive of a change we're really talking about.
Revolutionize. That is a strong word. I mean, what does that actually look like in practice?
Bob: Well, it's the difference [00:01:00] between, constantly putting out fires and actually stopping them before they even start built. Imagine having systems that can identify and then mitigate risks before they even become a problem.
Alice: Okay, so Less reacting and way more preempting that has got to be music to a risk manager's ears. But how does it even work? I mean, McKinsey gets into some pretty specific use cases, right?
Bob: They do, and they're actually surprisingly practical. One of the big ones is this idea of a virtual expert.
Imagine having an AI that has basically read every single regulation, every internal policy, every little piece of market data, and can instantly answer your questions about Potential risks.
Alice: So, like a 24 7 team of, like, super analysts at your fingertips? Exactly. I can see the appeal. No more all nighters digging through those massive compliance manuals.
So what else is there?
Bob: Well, there's manual process automation. And that's where generative AI takes over those super repetitive tasks that suck up so much time for risk and compliance teams like generating reports or flagging suspicious activity, [00:02:00] even drafting credit memos,
Alice: right. So it frees up the human experts for the stuff AI can't do yet, like those judgment calls where you need a nuanced understanding of the situation.
Bob: Exactly. Get this, generative AI can actually help write and update the code that powers a bank's risk systems.
Alice: So it's not just analyzing data, it's actually building and improving the systems themselves. That's wild. Okay, so we've got a handle on how generative AI can be used. What about where it can be used? Where in banking and finance does McKinsey see the biggest impact for this technology?
Bob: They really highlighted six key areas.
Regulatory compliance, financial crime, credit risk, modeling and data analytics, cyber risk, and, increasingly important, climate risk.
Alice: And each one has its own challenges and opportunities where generative AI could really shake things up.
Bob: Absolutely.
Alice: So let's break those down a bit. Starting with regulatory compliance, it feels like banks are always playing catch up with the latest rules and regulations.
Bob: Oh, it's a never ending game trying to stay ahead of the curve. But imagine if you could take [00:03:00] all those new regulations, feed them into a system that could instantly analyze them, pinpoint any potential conflicts, even suggest changes to your policies and procedures.
Alice: That brings us to financial crime, which honestly seems like it's evolving faster than ever these days.
Bob: Absolutely. And that's where the real time analysis capabilities of generative AI are so valuable. Imagine being able to sift through massive amounts of data transactions and flag anything that looks suspicious, your potential money laundering fraud, even terrorist financing as it's happening.
Alice: Having a digital detective on the case, 24 7. And it's constantly learning and adapting, so it's only going to get better at spotting those needles in the haystack.
Bob: Exactly like a detective who's also a master of disguise. Now let's talk about credit risk. That's where this virtual expert we talked about can really shine.
Alice: Okay, walk me through it. How would a loan officer actually use this day to day?
Bob: So imagine this, you're looking at a loan application, right? Instead of pulling credit [00:04:00] reports, verifying income, trying to connect all the dots yourself, you have this AI that can look at the applicant's entire financial history transactions, even news articles, social media, all in a flash.
Alice: Wow, talk about a complete picture that would take human analysts days, weeks to put together.
Bob: Exactly. And then the AI takes all that and gives you a risk assessment, gives the loan officer a much better basis for making a decision.
Alice: And that could seriously speed up the whole lending process, maybe even make it more equitable since it's looking at so many different factors.
That's a really interesting point about equity. We should definitely circle back to that because I feel like AI has the potential to go either way with that. But we've got a few more areas to cover.
Bob: Yeah, for sure.
Alice: What about modeling and data analytics? That one sounds a little more behind the scenes, you know?
It
Bob: is, but it's really the heart of good risk management. And this is where generative AI can help banks build and refine the really complex models they use to assess all kinds of risks. Market fluctuations, [00:05:00] credit defaults, you name it.
Alice: So fine tuning the bank's risk radar,
Bob: exactly. And it can even automate the process of checking how well those models are performing, flagging any potential issues, like if a model is getting outdated or if there's new data that needs to be considered.
Alice: So it's like having a whole team of data scientists working 24 7 to make sure the bank's risk management is always running in tip top shape. Okay, what about cyber risk? I feel like these days you can't talk about banking without talking about cyber security.
Bob: It's true. Cyberattacks are a constant threat.
No. Banks are a prime target. But here's how generative AI can help you. Imagine using it to not only find vulnerabilities in your systems, but to actually simulate cyberattacks. See where your defenses hold up and where they might crumble.
Alice: So you're basically training your defenses against every possible attack.
Bob: Yeah, in a way you're using AI to think like the attacker and build more resilient systems from the ground up.
Alice: That's incredible. And then there's climate risk, which I imagine is only going to become more and more important for banks to figure out.
Bob: [00:06:00] Absolutely. Climate change presents all kinds of risks for banks and their clients.
But this is another area where AI can be super valuable.
Alice: How so?
Bob: Think about it, assessing a company's exposure to climate change. That means looking at tons of data, their carbon emissions, their supply chains, their physical locations, how vulnerable they are to things like extreme weather. It's a huge undertaking.
Alice: Yeah, not exactly something most banks are equipped to handle on a large scale right now.
Bob: Exactly. But generative AI can help them analyze all that data, connect the dots, Identify the key climate risks and opportunities, and then use that information to make smarter lending decisions, develop new financial products, even work with our clients on climate related stuff.
Alice: It's like having a crystal ball that can see into the future of climate change and how it'll impact their business.
Bob: In a way, yeah, it's about using AI to make sense of really complex information and make better decisions when things are uncertain.
Alice: Okay. So we've talked about the upsides, which honestly sound pretty amazing, but let's be real.
There have to be some downsides [00:07:00] too. I mean, no technology is perfect.
Bob: Absolutely. And the McKinsey report doesn't shy away from that at all. They actually highlight eight key risks that banks need to be aware of when it comes to generative AI.
Alice: Okay. Let's hear them. What are the potential pitfalls? Okay, eight risks.
Lay it on me. What's the first one banks need to watch out for?
Bob: ~~Well, ~~remember how we were talking about AI may be making lending more equitable?
Alice: Yeah.
Bob: So that's actually where one of the biggest risks comes, and McKinsey calls it impaired fairness. Because these AI models are trained on huge amounts of data.
But if that data has existing, ~~you know, ~~biases, let's say from past lending practices that were discriminatory. The AI could actually pick up on those biases and make them even worse.
Alice: So instead of fixing the problem, it makes it worse, even if it's not on purpose.
Bob: Exactly. It's not as easy as just, you know, turning on the AI and letting it run wild.
You need people to keep an eye on it, make sure it's actually making things fair and not the opposite. And it's not just fairness. There's the whole intellectual property question, if this AI is creating new stuff like code [00:08:00] or marketing materials, who owns the rights to that, especially if it's based on existing copyrighted material.
Alice: ~~Yeah. ~~That sounds like a legal mess waiting to happen.
Bob: It really is. And it gets even more complicated when you think about privacy. We're talking about AI that can create super realistic personalized content, which could be great for customer service, but it also brings up big questions about data security.
~~Could this be used in ways that violate? ~~
Alice: Yeah, it's a big responsibility, it feels like, with any new technology, especially something this powerful, there's always the risk of it being used for, ~~you know, ~~bad stuff too.
Bob: Exactly, and that's another risk McKinsey talks about, malicious use. ~~As much as ~~this tech can ~~be used to manage risk, it can also ~~be used by bad actors to create deepfakes, spread misinformation, or even come up with completely new ways to commit financial crimes.
Alice: So it all comes back to who's controlling the technology and what they decide to do with it. What else should banks be on the lookout for?
Bob: Well, even if everyone's intentions are good, there are technical risks too.
Alice: Yeah.
Bob: These models are so complex, even the people who design them don't always fully understand why they make a particular decision.
[00:09:00] Right. And that lack of explainability can be a real problem, especially in something like risk management, where being able to justify a decision, especially if something goes wrong, is so important.
Alice: It's hard to trust a system if you have no idea how it actually works.
Bob: Exactly. And then there are the more practical risks, like what if a bank becomes too reliant on AI and then something goes wrong?
A technical glitch, bad data, it could be a disaster for their operations and their reputation.
Alice: ~~Yeah. Talk about putting all your eggs in one very, very high tech basket. ~~Yeah. Not exactly what you'd call good risk management.
Bob: Right. Which brings us to the last risk McKinsey highlights. Third party risk. As banks use more of these generative AI tools, ~~they're also depending more on third party vendors, so ~~they need to be super careful about who they're working with.
Make sure those vendors have top notch security and ethical guidelines.
Alice: Because one weak link in the chain can take down the whole system?
Bob: Exactly.
Alice: Okay, so we've covered a lot of ground here, both the good and the bad, all the amazing opportunities and the very real risks. So what's McKinsey's advice to banks?
How can they actually approach this technology strategically and [00:10:00] responsibly?
Bob: Will they lay out a seven point plan? ~~And it really emphasizes being proactive and thoughtful about this whole thing. ~~
Alice: ~~Okay, I'm all ears. ~~What's step one?
Bob: Focus on impact. Don't try to do everything at once. Find a few key areas where generative AI can make a real difference.
Maybe areas with high risk or where it can free up your human experts to focus on more complex tasks and prove the value before you go all in. Start small and scale up. Exactly. And then you need to build that generative AI ecosystem and that goes way beyond just the tech itself. It's about having the right data management systems, attracting the right people, building a culture that's open to experimenting and learning.
Alice: So creating the right environment for success.
Bob: Exactly. And that also means embracing the cloud ~~banks need that massive computing power that the cloud offers ~~to process the huge amounts of data involved in generative AI.
Alice: ~~So flexibility is key. ~~What else?
Bob: Well, remember how we talked about bias in the data ~~point 4 is all about prioritizing data quality ~~generative AI is only as good as the data it's trained on.
Alice: Garbage in, garbage out, right?
Bob: Exactly. That's why developing new talent models is so important. Banks need to train their existing employees, but also be ready to bring in new talent with specialized skills in AI data science, [00:11:00] machine learning, all of that.
Alice: So investing in the future of the field.
Bob: Exactly. ~~And because this tech really touches every part of how a bank works, fostering a culture of collaboration is essential. ~~Risk management teams need to be working closely with IT, with data scientists, with the business side. Everyone needs to be on the same page.
Alice: Break down those silos.
Bob: Exactly. And then finally, and this is crucial, don't forget the human element, as amazing as generative AI, is it's not a magic solution. Human judgment, expertise, ethical considerations, those are still essential.
Alice: So AI is a tool, not a replacement.
Bob: Exactly. It's about finding that balance.
Alice: Yeah. This has been a fascinating deep dive and it makes you really think, if AI can be used to manage risk, could it also be used to create completely new risks that we haven't even imagined yet? Something to ponder.
Bob: Now that is a question and one I think we'll be wrestling with for a long time to come.
Alice: That's all the time we have for today's deep dive, ~~but keep those brains buzzing and ~~we'll catch you next time.