🍿AI highlights from this week (2/3/23)
Google invests $300M in OpenAI competitor plus more
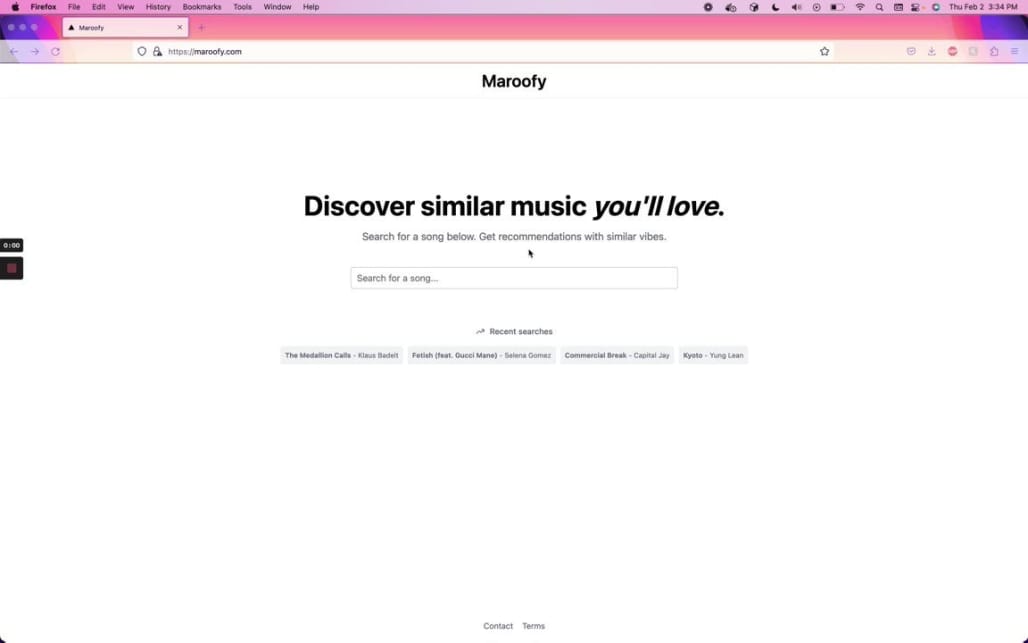
Hi readers,
Here are my highlights from the last week in AI, including a music recommendation product and Google investing $300M in an OpenAI competitor.
P.S. Don’t forget to hit subscribe if you’re new to AI and want to learn more about the space.
The Best
Here’s the best of what I read this week:
1/ Maroofy: Search for songs that sound like this one
Maroofy is a song discovery app created by @Subby_tech, an AI engineer who has been launching new AI-based apps regularly on Twitter! The app is a search engine that lets you find songs that are sonically similar to the one you enter and is trained of over 140,000 sounds from iTunes.
✨ Introducing Maroofy
— Subhash Ramesh (@subby_tech) 11:45 PM ∙ Feb 2, 2023
Search for any song & it'll use the song's audio to find similar-sounding music.
🧠 Powered by an AI model trained on 120M+ songs, for 🔥 recommendations.
@_buildspace @fdotinc @FarzaTV
🔊 Demo + link below!
You may be familiar with Spotify’s song recommendations that use meta-data about songs and a collaborative filtering1 process that recommends songs based on users with similar tastes to you. Maroofy, on the other hand, uses the song’s audio in its training. The songs it produces have a similar rhythm, instrument arrangements, and overall sonic qualities. I found that Maroofy performed better for songs by well-known artists like the Beatles but came with no recommendations for newer artists like Fred again.
Try it out yourself here: Maroofy.com
2/ OpenAI launches new AI classifier to combat “AIgiarsim”
OpenAI announced last week that they had developed a new tool to identify AI-written content. This was likely in response to the growing concern, especially from educators, that AI would be used by students to “cheat”.
We’re developing a new tool to help distinguish between AI-written and human-written text. We’re releasing an initial version to collect feedback and hope to share improved methods in the future.
— OpenAI (@OpenAI) 6:10 PM ∙ Jan 31, 2023
Some educators however are embracing AI including a Wharton Business School professor recently interviewed by NPR who is requiring his student to use ChatGPT
This year, Mollick is not only allowing his students to use ChatGPT, they are required to. And he has formally adopted an A.I. policy into his syllabus for the first time.
He teaches classes in entrepreneurship and innovation, and said the early indications were the move was going great.
"The truth is, I probably couldn't have stopped them even if I didn't require it," Mollick said.
Many have compared the use of ChatGPT as similar to allowing Math students to use calculators in exams, but I think that’s a misleading analogy. Here’s a couple reasons why:
- A Calculator can be used to answer a question, but it requires a human to understand the question and input the correct formula. ChatGPT, on the other hand can answer a question verbatim, removing the need for a student even to understand the question itself.
- A Calculator is programmed to provide mathematically correct answers deterministically. ChatGPT is trying to probabilistically predict the answer to a question with no guarantee of being correct. In other words a student can rely on a calculator to get the answer right. Still, if they don’t understand the subject matter sufficiently, they could easily be tricked by ChatGPT into thinking an incorrect answer is correct!
I would therefore liken ChatGPT to be more like “Phone a friend” in the gameshow Who wants to be a millionaire? Your very knowledgeable friend ChatGPT probably knows the answer to the $1M question when you call them up, but you’re putting your trust in them, not the truth!
3/ OpenAI improves ChatGPT maths skills
Speaking of ChatGPT trying to do maths, last week, OpenAI released an update to ChatGPT to improve its “factuality and mathematical capabilities”:
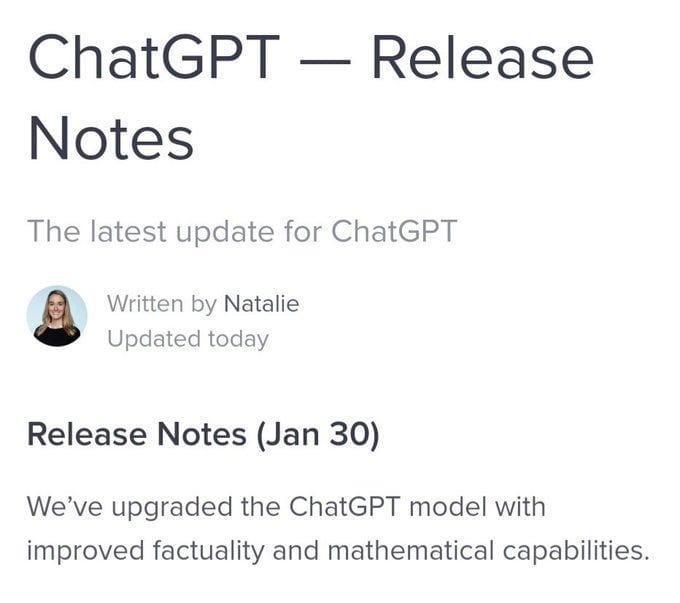
Of course, it didn’t take long for the netizens of Twitter to put this claim to the test… 😬
@tunguz Needs work on that maths though...
— Bryan Nelson 🇿🇦🇳🇱 🏊🚴🏃 (@btnelson) 9:52 PM ∙ Jan 30, 2023
@tunguz Nope. Tried just now. ChatGPT is still a husband.
— ANIMOL (@animolofficial) 2:06 AM ∙ Jan 31, 2023
But you may be wondering why ChatGPT is so bad at math, after all, didn’t we solve this problem with calculators decades ago? ChatGPT is a large-scale language model (LLM), which is trained on massive amounts of text information to be good at predicting how to respond to your answer. It now has logical abilities to do math other than what it has learned from reading text. It’s impossible for an LLM can learn concepts like how addition and subtraction in the logical way that we do. What they can learn is the probability of what number to say next in the phrase “2+4=”, but as you can see in the second example, because ChatGPT is trained on human feedback, it is just as happy to agree with you that 2+4=8!
4/ Google $300M invests in OpenAI competitor Anthropic
Hot off the press, Google has invested an eye-watering $300M for a 10% stake in Anthropic, a San Francisco-based AI startup founded by researchers from OpenAI. Anthropic is working on a ChatGPT competitor Claude, currently in private beta testing. This detail in the Financial Times article covering the fundraising stood out to me:
Anthropic was formed in 2021 when a group of researchers led by Dario Amodei left OpenAI after a disagreement over the company’s direction. They were concerned that Microsoft’s first investment in OpenAI would set it on a more commercial path and detract from its original focus on the safety of advanced AI.
That concern from Anthropic’s founders turned out to be well founded as OpenAI and Microsoft are planning to add many of ChatGPT’s capabilities into Microsoft’s software suite, including Teams, according to Satya Nadella, Microfost’s CEO:
We’re bringing the power of large language models, including OpenAI’s GPT, to Teams Premium, as we make meetings more intelligent, personalized, and protected.
— Satya Nadella (@satyanadella) 11:13 PM ∙ Feb 1, 2023
Meanwhile Claude isn’t available to the public today, but you can get a sense of how it’s different from ChatGPT in this article by Scale comparing the two:
"Meet Claude: @AnthropicAI's Rival to ChatGPT"
— Riley Goodside (@goodside) 2:06 AM ∙ Jan 18, 2023
Through 40 screenshot examples, we explore the talents and limitations of ChatGPT's first real competitor.
My first writing for @scale_AI, coauthored with @spencerpapay.
5/ The Consumer AI space is heating up
According to an article by Reuters, ChatGPT is estimated to have 100 million active users, an extraordinary feat for a product that is less than two months old!
From Reuters:
The report, citing data from analytics firm Similarweb, said an average of about 13 million unique visitors had used ChatGPT per day in January, more than double the levels of December.
"In 20 years following the internet space, we cannot recall a faster ramp in a consumer internet app," UBS analysts wrote in the note.
It took TikTok about nine months after its global launch to reach 100 million users and Instagram 2-1/2 years, according to data from Sensor Tower.
ChatGPT might be a sign of more product innovation powered by AI coming to the consumer space, which hasn’t got much love from startup founders in the last few, opting to focus on b2b products instead. This week former Instagram founders Kevin Seistrom and Mikey Kreig also announced a new consumer-focused AI Newsreader, Artifact. Here’s how the app will work according to The Verge who broke the story:
The simplest way to understand Artifact is as a kind of TikTok for text, though you might also call it Google Reader reborn as a mobile app or maybe even a surprise attack on Twitter. The app opens to a feed of popular articles chosen from a curated list of publishers ranging from leading news organizations like The New York Times to small-scale blogs about niche topics. Tap on articles that interest you, and Artifact will serve you similar posts and stories in the future, just as watching videos on TikTok’s For You page tunes its algorithm over time.
I found this little tidbit in the article to be interesting too:
The breakthrough that enabled Artifact was the transformer, which Google invented in 2017. It offers a mechanism for systems to understand language using far fewer inputs than had previously been required.
It seems then that Artifact is using Transformers2 to provide recommendations to users though the article didn't state precisely how. My guess is that they are training a model to predict what a user will want to read next based on what many readers have previously read.
The experience sounds very similar to the Google News app, which uses AI to make recommendations too. However, I’m sure Artifact will be much more polished and visually appealing, given the product’s founders created Instagram. You can sign-up the waitlist for Artifact here: https://artifact.news
The Rest…
Here’s everything else I read this week:
Unconfirmed screenshots of the "New Bing" with OpenAI GPT integration from @Owen_Yin
— AI Breakfast (@AiBreakfast) 4:13 AM ∙ Feb 4, 2023
-Chat window replaces search bar
-Conversational UI
-Citations
🚨ɴᴇᴡ ꜰᴇᴀᴛᴜʀᴇ ᴀʟᴇʀᴛ🚨
— Des Traynor (@destraynor) 5:45 PM ∙ Jan 31, 2023
The day ChatGPT launched our ML team got straight to work, asking how it could make @intercom better at Customer Service.
Today we're announcing our first wave of features...
One of the most interesting ideas I've come across is the hybrid neural network for computer vision. ❓❓❓
— Devansh: Machine Learning Made Simple (@Machine01776819) 6:02 PM ∙ Feb 3, 2023
Check out this thread to find out more about them and why you might want to use them instead of Convolutional Neural Networks for your Image Classification Tasks 👇
Okay #stablememefusion @fastdotai meme thread. Here we go.
— Jupyter Meowbooks (@untitled01ipynb) 12:07 PM ∙ Oct 26, 2022
Starting with lecture 9. I'll scribble meme ideas as I listen on x2 🙏
Finally, in case you missed it, I made a comparison of Google’s lastest AI models against the competition:
That’s all for this week!
Thanks for reading The Hitchhikers Guide to AI! Subscribe for free to receive new posts and support my work.
Collaborative filtering is a technique used by recommendation systems to make user suggestions based on their past behavior. It works by analyzing patterns in the behavior of multiple users and then using that information to make recommendations to other users with similar interests. For example, suppose a user frequently watches romantic comedies. In that case, the system may recommend other romantic comedies to them based on the viewing patterns of other users who have shown an interest in that genre. The idea is that people with similar tastes in movies, books, music, etc. are more likely to enjoy similar things in the future. Hence, the system tries to identify these patterns and make personalized recommendations. ↩
In 2017 Google’s AI researchers published a paper, “Attention is All You Need” where they proposed a new neural network architecture called the Transformer. This architecture created a step change progress in AI which enabled Large-scale Language Models like ChatGPT and Generative AI like Stable Diffusion. You can learn more about transformers in my part 3 of my series on the origins of deep learning: ↩
🤓A Deep Dive into Deep Learning: Part 3Hi Readers! Thank you for subscribing to my newsletter. Here’s the final part of my deep dive into the origins of deep learning. In case you missed it, here are Part 1 and Part 2. The field of deep learning is filled with lots of jargon. When you see the 🤓 emoji, that’s where I go a