AI: Don’t believe the hype? 🤖 🚀
Why AI is getting so much attention and why you should care
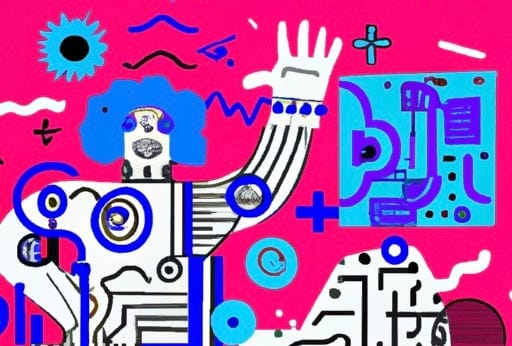
Hello readers!
In my first newsletter I wanted to share why I’m excited about AI and why I think it’s going to completely change the way we build products and interface with software. I’ll cover why there was so much excitement around AI in the last year, what the major advancements were, and what enabled them. I’ll also share some predictions for where we are headed in 2023. This first post is really aimed at folks that are completely new to AI and want to get up to speed with the latest developments. If you’re more interested in getting into the technical side of AI, I’ll be going over that in future posts!
If you want to learn more about who I am and why I got into AI check out my Welcome post.
P.S. Don’t forget to subscribe if you want more content about AI and are new to the space.
Why did AI get so exciting in 2022?
AI is a pretty broad term that covers many different types of technologies from machine learning to computer vision, self driving cars to chatbots. Most of these technologies have been in development for decades. What made 2022 an exciting year for AI was the developments made in two areas of AI in particular which together have been grouped into Generative AI:
Large Language Models with generative capabilities1 (e.g. Open AI’s GTP3 and ChatGPT, Google’s LaMBDA)
Diffusion Models2 (e.g. Stable Diffusion, MidJourney, and Open AI’s Dall-E)
Unlike previous advancements in AI which have either been too specialized to be broadly appreciated by most people (e.g. models that detect tumors in X-rays) or have fallen far short of expectations (e.g. voice assistants and self-driving cars), the recent breakthroughs in both LLMs and Diffusion have three things in common that set them apart:
1/ Broadly accessible
AI’s breakthrough moment happened this year because a number of different AI models became publicly accessible to everyday consumers. Anyone can go to chat.openai.com and within seconds start interacting with Open AI’s ChatGPT chatbot. This created instant buzz and excitement as people shared their chat experiences with each other and the product grew virally.
ChatGPT launched on wednesday. today it crossed 1 million users!
— Sam Altman (@sama) 7:35 AM ∙ Dec 5, 2022
Similarly the text-to-image generators Dall-E 2, Stable Diffusion and Midjourney all became publicly available and as folks created images and shared them on social media, it inspired others to follow suit, creating a whole new genre of AI generated Art.

1/ Indistinguishable from magic
“Any sufficiently advanced technology is indistinguishable from magic.” - Arthur C. Clarke
Unlike it’s predecessors (e.g. Google Assistant, Echo, Siri), ChatGPT is really the first time an AI assistant truly seems like it could pass the Turing Test. There have been many impressive examples of ChatGPT in action and if you haven’t tried it yourself you should. ChatGPT successfully wrote a blog post for me and turned it into a twitter thread, gave me a recipe for pancakes that tasted delicious and helped me pick a Christmas present for my wife! What truly impressed me though is ChatGPT’s ability to be “creative”. For example, my aunts often shares poems they writes in in our family Whatsapp thread, so in an effort to showcase the power of AI I asked ChatGPT to write a poem relevant to my family. Here’s what it came up with…



Meanwhile, Dall-E gives anyone the power to create images from their imagination just by describing them. A friend recently created an illustrated children’s book and published it in a weekend using a combination of ChatGPT and Generative AI!
I spent the weekend playing with ChatGPT, MidJourney, and other AI tools… and by combining all of them, published a children’s book co-written and illustrated by AI!
— Ammaar Reshi (@ammaar) 6:35 PM ∙ Dec 9, 2022
Here’s how! 🧵
These examples have captured people’s imaginations and got people excited about AI in a way that we haven’t witnessed in a long time. It feels like the sci-fi version of AI that we were all promised has all of a sudden come closer to fruition.
3/ Open sourced or available via API
Of course, many of these models are open sourced or available via API so other developers can easily build their own products on top of it, further broadening its reach. For example, Lensa added the ability to easily generate AI based profile pictures using Stable Diffusion, which went viral. Afterall who doesn’t want to spend $10 to get a more attractive and cool looking profile pic?!
The growth of the Lensa app is something to behold.
— ✮ Mr. Mugen ✮ (@iam_mugen) 8:54 PM ∙ Dec 8, 2022
Doing over $1MM in revenue 🤯
#AiIsTheFUTURE
Meanwhile a good counterexample of accessibility is that even though Google created LaMBDA, an LLM that is supposedly superior to GTP3, because it wasn’t publicly available there wasn’t any hype around it except for the Google researcher who thought it was sentient3.
What made these AI advancements possible?
There have been many developments in AI that have led to ChatGPT, GPT-3, and DALL-E, as well as other generative AI models. Some of the major ones include:
Deep learning: One of the key technologies that has enabled the development of these models is deep learning, which refers to the use of neural networks with many layers to learn patterns and features from data. This has allowed models to learn complex representations of language and other types of data, and has been instrumental in the development of natural language processing (NLP) and other AI tasks.
Large-scale data and computing resources: Another important factor has been the availability of large amounts of data and computing resources, which have allowed researchers to train and fine-tune these models on a very large scale. This has been made possible by advances in hardware and software, as well as the emergence of cloud computing platforms.
Unsupervised learning: Many of these models are trained using unsupervised learning, which means that they can learn from data without being explicitly told what to do. This has allowed them to learn to perform tasks like translation and summarization without the need for large amounts of labeled data.
Transformer architecture: The transformer architecture4, which was introduced in 2017, has also played a key role in the development of these models. It is a type of neural network architecture that is particularly well-suited for NLP tasks, and has been widely used in the development of language models like GPT-3.
Pre-training and fine-tuning: Another important trend has been the use of pre-training and fine-tuning, which involves training a large model on a large dataset and then fine-tuning it on a smaller dataset for a specific task. This has allowed models like GPT-3 to perform a wide range of tasks with relatively little task-specific training data.
Reinforcement Learning from Human Feedback (RLHF): A type of machine learning approach that uses reinforcement learning (RL) principles in combination with human feedback to train AI systems. In RLHF, a machine learning model is trained to take actions in an environment in order to maximize a reward signal. The model receives feedback in the form of rewards or penalties for its actions, which it uses to adjust its behavior and improve its performance over time. This is how ChatGPT became so good at conversation.
I plan to cover many of the above areas in detail in dedicated posts in the future as I learn more about AI so look out for those.
What’s next?
We’re likely going to see a lot more momentum around AI in 2023, much of it built on the progress in LLMs and Generative AI from last year. A lot of the advancements in the last year were very much at the research and testing phase, for example ChatGPT was launched by OpenAI as a “Free Research Preview”. What I’m most excited about over the next year is how these foundational models will be used to build compelling products for end customers, how they will become actual businesses and how those businesses will differentiate from each other meaningfully.
Here’s a few specific areas to keep an eye on in 2023:
GPT-4: Bigger, Better, More Powerful?
Currently, GPT-3 has 175 billion parameters, which is 10x faster than any of its closest competitors. GPT-4 is rumored be about 100 trillion parameters.
— AI Breakfast (@AiBreakfast) 3:53 PM ∙ Nov 21, 2022
OpenAI launched GPT 3.5 via their API in December and there’s lots of anticipation around GPT 4 which will launch early this year. GPT-4 is rumored to have approximately 100 trillion parameters (100X more than GPT-3), potentially making it as complex as the human brain. The number of parameters in an artificial intelligence model does not necessarily correlate with its performance, but rather is just one factor that can impact performance. GPT-4 is expected to have a wide range of applications, including answering questions, summarizing text, translating languages, and generating code5. There’s a lot of hype around GPT-4 and we won’t really know if it will live up to it until it launches.
Generative AI Models for video and audio and other formats
We will likely see breakthroughs in generative AI for content that has a temporal component, i.e. video and audio. Harmonyai is an open source project that spun out from Stable Diffusion that I’ve been following closely in the audio space. They have already developed a model that can generate audio by fine training on existing audio but no-one has cracked text-to-audio yet. The closest project is Riffusion which takes a pretty interesting approach of first converting audio in images via Spectrogram6, then using stable diffusion to do text-image generation and then converting the image, also spectrogram, back into audio!
Last year both Meta and Google announced text-to-video AI systems in 2022 but they aren’t yet widely available and very much at the research stage. It will be interesting to see if a startup like OpenAI makes a more accessible version in 2023.
Specialized writing products built on generalized models
We’re going to see lots of new products emerge over the next few years that take the foundational models like GPT-3 and apply them to a specific vertical content creation task, packaged in a product that is designed for a particular target customer. A great example of this is Jasper, the AI powered copywriting product that uses GPT-3 to help businesses generate content e.g. for paid Ad copy, blog posts, marketing copy, social media posts, sales emails).
Here are a few other use cases we will likely see products built around:
- Email autocomplete / auto responder
- Outbound sales
- Recruiter emails
- Internal Documentation and Wikis
- Influencer marketing content
- Customer support automation
Anywhere that a human is communicating information in a repetitive manner or where there is need to summarize content, a model like GPT can be very effective to improve productivity. This is probably where we will see the biggest change in how we are used to interfacing with software over the next few years. Think about all the mundane writing tasks you do and how you can speed them up.
As an Example, if you are a PM you probably want to spend more of your time thinking about strategy, coming up with potential solutions with your team, unblocking execution and talking to customers. But you actually spend a lot of your time painstakingly writing PRDs, updates to stakeholders etc. AI products like ChatGPT can help you do this a lot faster giving you back more time to do higher leverage work7.
I know friends that are already using ChatGPT to write product requirements, quarterly exec updates etc. In fact I used ChatGPT to help write a lot of this blog post!
AI Middleware
Today’s AI models are either available as open source to deploy yourself or as fairly rudimentary APIs requiring lots of prompt engineering. I believe there will likely be a new layer of abstraction built on top of existing AI models that make it much easier for developers to build more specialized apps and do some of the prompt engineering / fine tuning work for you as well as making it easier to tune parameters of the models. Even more powerful than this is if AI itself powered the middleware, for example teaching the model that it can write specific code for a use case and then executing that code on behalf of the model in order to solve the problem. An example of this would be to have a specialized middleware for GPT3 that trains it on how to fetch a website and extract the content of that website from HTML. This middleware could then be used by other products for any number of use cases e.g. summarizing news articles.
AI Assistants / Chat bots 2.0
ChatGPT, but in iMessage
— Soren Iverson (@soren_iverson) 3:46 PM ∙ Jan 3, 2023
This is an area I’m particularly excited about and have already seem some compelling prototypes of. The idea is that instead of an end user interfacing directly with existing products like Search, News, Calendars, Email, etc. the user interacts with conversational AI like Chat GPT which is integrated with these products via API. So, let’s say you wanted the latest news on Inflation in the US, your AI assistant will first interpret that you want news, then make an API call to an news provider, then summarize that news for you. This was the promise of Google Assistant / Siri / Echo but my prediction we will see more interactive and human-like version of this, probably in Chatbot form. Imagine if you could ask ChatGPT to create your grocery list based on a recipe you want to make and then order it on Instacart! Maybe I’m just dreaming…
Differentiation
This will likely be a big topic in 2023 as AI startups start raising funding and investors try to work out if their ideas are truly defensible. The biggest question will likely be around whether the moat a startups build will be in the model, the data or the product experience. Nathan Lambert makes the argument in Predicting Machine Learning Moats that AI models will be too easy to replace and copy, by fine tuning or even training on existing models via a process call model distillation8. Lambert predicts that differentiation will come from emergent behavior, whereby a model reaches a certain data threshold and starts achieving nonlinear growth in performance i.e. Data becomes the moat. I have confess I don’t know enough about AI yet to understand this fully but my two cents is that we will see the most differentiation in the product experience and that models themselves and access to models will become commoditized. This will especially become true if Google launches its own developer version of LaMBDA and makes it available via it’s cloud services.
Why read this newsletter?
Wow this was a longer first post than I was planning but if you got this far I hope you at least found the information useful! As you can see there’s a lot to be excited about in the AI space this year and plenty of substance to all the hype. I created this newsletter mostly as a forcing function for me to write down my thoughts about the space as I dig deeper, summarize what I’m learning so it sticks and help other folks who are also approaching AI for the first time and might be intimidated. My plan is go deeper into the technical side next because that’s what I’m most curious about so if you want to understand how all this AI stuff works, smash that subscribe button! I’ll do my best to make the content as approachable and non-technical as I can. I’ll also be sharing any useful content I’ve read / listened to along the way.
With that in mind, I recommend checking out the following newsletters to stay up-to-date on the space:
- - Commentary on AI related technology and business
- - Weekly digest of AI news with a great 2022 roundup.
- - In depth articles research and trends in AI
- - Daily bite-sized AI updates and links.
If you’re a Ben Thompson fan, his latest podcast episodes interviewing Daniel Gross and Nat Friedman was also a really great primer on the recent AI developments that I enjoyed listening to:
That’s it for my first post. Please don’t forget to hit Subscribe if you haven’t already!
Thanks for reading The Hitchhikers Guide to AI. Subscribe for free to weekly content for builders, founders and product managers new to AI.
A large language model is a machine learning model that is trained to predict the next word in a sequence of words. It is called "large" because it is trained on a large dataset, typically made up of billions of words. The model is able to learn the statistical patterns and relationships between words and their meanings, allowing it to generate human-like text when given a prompt. Large language models have been used for a variety of tasks, such as translation, summarization, and question answering. ↩
Diffusion models were an expected effective way to generate images from text. They work by destroying images into noise and then learning how to rebuild those images back be de-noising them. While Dall-E was the first Diffusion model to be publicly released it was not open source. Stable Diffusion, created by the researchers and engineers from Stability AI, CompVis, and LAION, was then released as open source alternative and that was better than Dall-E and available for anyone to fine-tune and change!
You can learn more about Stable Diffusion here: Stable Diffusion: Best Open Source Version of DALL·E 2 ↩
Google Sidelines Engineer Who Claims Its A.I. Is Sentient - NY Times ↩
The Transformer Architecture was defined in "Attention is All You Need", a research paper published in 2017 that introduced the Transformer, a neural network architecture for natural language processing tasks such as machine translation. The paper was written by Vaswani et al. and was published in the proceedings of the 31st International Conference on Neural Information Processing Systems (NIPS).
The authors of the paper demonstrated that the Transformer architecture outperformed previous state-of-the-art models on a number of natural language processing tasks, including machine translation, language modeling, and summarization. The success of the Transformer architecture has led to its widespread adoption in the field of natural language processing. ↩
GPT-4: Facts, Rumors and Expectations about next-gen AI model ↩
A spectrogram is a visual representation of the spectrum of frequencies of a sound or other signal as it varies with time. It can be thought of as an "audio image" or a "visual snapshot" of the sound. The horizontal axis of the spectrogram represents time, while the vertical axis represents frequency. The intensity or brightness of the image at any particular point is determined by the strength or amplitude of the frequency at that point in time. ↩
Shreyas Doshi has a great framework for leveraging hour time as a PM. You can read more about it here: LNO Framework for Product Managers ↩
“When a model like GPT3 is opened up via an API, users can generate numerous outputs associated with prompts, and use them to fine-tune a model of a similar architecture. While this isn't perfect, it's expected to create a model of similar performance (and is conceptually much simpler than doing so from scratch). ChatGPT, for example, is currently free, so people could be doing this in the background. If a typical NLP training set has 1 billion training tokens, you would burn about $ 10 million of computation from OpenAI/Microsoft and be able to train something like chatGPT” - Predicting machine learning moats by Nathan Lambert. ↩