AI and the Movies
A look at how AI might impact film making and the movie industry
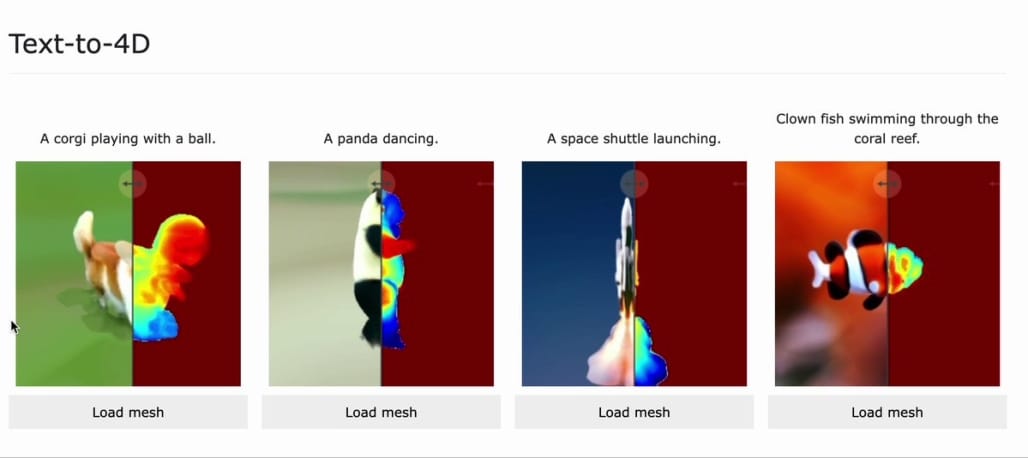
Hey Hitchhikers!
First, a huge welcome to the 130 new subscribers that hitched a ride with us this week! In this post, I will continue on the theme of AI and entertainment. Last week I covered AI and the future of music. This week we’ll look at the latest advancements in generative video and discuss how AI could impact the future of moviegoing and video consumption.
Before we jump in, I would love your help in getting to my goal of 5,000 subscribers in June. We’re almost 70% of the way there already. All you need to do is share this newsletter with a friend!
The State of Generative Video
We last talked about generative video in this newsletter in February when I covered Google’s latest research in the space. Here’s a quick reminder of what state-of-the-art looked like just 3 months ago, quoting from that post:
Google shared that one of it’s next big areas of focus for AI research is Generative Video which is more complex because of the time component, as Jeff Dean shared:
“One of the next research challenges we are tackling is to create generative models for video that can produce high resolution, high quality, temporally consistent videos with a high level of controllability. This is a very challenging area because unlike images, where the challenge was to match the desired properties of the image with the generated pixels, with video there is the added dimension of time.”
In video generation, Google’s latest models are Imagen Video20 and Phenaki21. Both models are text-to-video, but Imagen Video uses Diffusion Models, whereas Phenaki uses Transformers. At a high level, Imagen Video works by using T5, a text-to-text Transformer by Google, to embed22
the input prompt say, "A cat floating through space" into numerical data. This numerical data is then used to condition a diffusion model23
to generate a video consistent with the prompt. This process is similar to image diffusion but uses a video diffusion model that Google published in June 2022. The initial output video is more like a storyboard of the final video, with just 16 frames of video at 3 frames per second verses 24 frames per second for a film. Two more models are then used to fill the video with additional frames and increase the overall resolution. The resulting videos are limited to about 5 seconds long:
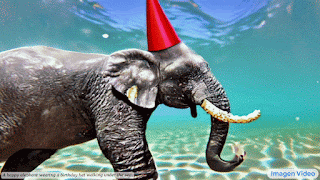
Phenaki, Google’s second generative video model, uses transformers to compress video to smaller bite-size pieces called “tokens” in machine learning parlance. These tokens could be a scene, a shot, or some action happening in the video, and they are compressed to be a more abstract representation than the raw video, making it easier for a model to process. A bi-directional transformer24 is then used to generate these video tokens based on a text description. The video tokens are then converted back into an actual video. According to Google, the model can generate variable-length videos, which makes it ideal for storytelling:
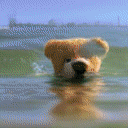
Google believes that both models can be used in combination to generate high-resolution long-form videos:
“It is possible to combine the Imagen Video and Phenaki models to benefit from both the high-resolution individual frames from Imagen and the long-form videos from Phenaki. The most straightforward way to do this is to use Imagen Video to handle super-resolution of short video segments, while relying on the auto-regressive Phenaki model to generate the long-timescale video information.”
Text-to-video generation is still in its nascent stages, and Google’s research does seem to be ahead further along than anyone else in the AI field, which makes it difficult to make any side-by-side comparisons. Meta did recently release their text-to-4d model MAV3D25, which you could imagine being used for Pixar-style animated video, but it doesn’t have the same photo realistic quality that Imagen Video or Phenaki do:
MAV3D (Make-A-Video3D) - a method for generating three-dimensional dynamic scenes from text descriptions. Using a 4D dynamic Neural Radiance Field (NeRF).
— Ben Tossell (@bentossell) 10:33 AM ∙ Jan 27, 2023
project page: make-a-video3d.github.io
arXiv: arxiv.org/abs/2301.11280…
A group of researchers from the University of Singapore also recently published Tune-A-Video26, a text-to-video model that, unlike its predecessors, is only trained onone text-videoexample to learn a particular scenario (e.g. A man surfing a wave) rather than a large-scale dataset of text-video pairs. Once the model is trained on a video of “A man skiing on snow,” it can then produce variations of “a panda” or “an astronaut on the moon”:
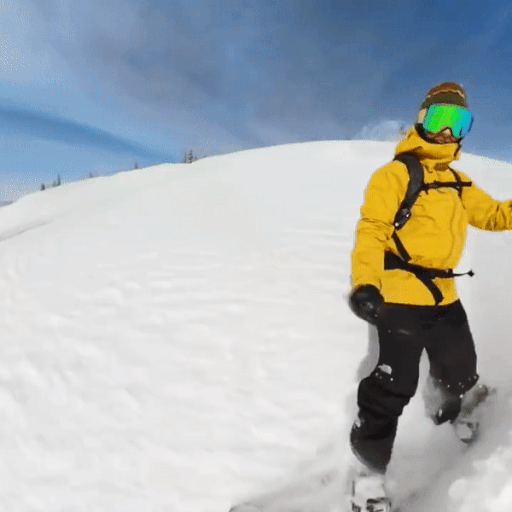
Since writing that article in February, we’ve seen several new advancements in how AI is being used to generate video:
1/ ControlNet
One of the most noteworthy is ControlNet, a neural network approach to customizing text-to-image diffusion models like StableDiffusion. For example you can provide ControlNet with an existing image of person, extract the post of that person, and then use the pose with StableDiffusion to create new images of people with the same pose.
While ControlNet wasn’t created for video when combined with tools like Dreambooth for fine-tuning Stable Diffusion models, Unreal Engine and existing VFX workflows, to create AI-generated animations. In this case, the pose of a character in each frame can be generated using ControlNet and Stable Diffusion, then combined together with additional tools. Here’s one of the first examples of this by Corridor Digital, published in early March:
2/ Wonder Dynamics’ AI-powered CGI
Also in March was Wonder Dynamics’ announcement of their new product Wonder Studio which uses AI to change the way motion capture is done by using AI to convert a person into CGI character instead of expensive motion capture rigs and green screens:
Since releasing Wonder Studio, several users have created demos of the technology, proving that the company could change the way modern CGI is done. Here’s a great example of an action sequence with hand-to-hand combat that was shared on Youtube:
By comparison, this scene would have taken many hundreds of hours to capture, animate and render using traditional CGI processes.
3/ Neural Radiance Fields
Neural Radiance Field1 or NeRF can create realistic 3D images or scenes using artificial intelligence. The magic of NeRF is that it can generate very realistic and high-quality 3D images even when the input images are imperfect or have missing information. This makes it a powerful tool in virtual reality, video games, and movies, where realistic 3D graphics are essential.
Remember that infamous scene in Enemy of the State they rotate CCTV footage to look at a different angle of what was dropped in Will Smith’s shopping bag?!
Well, that’s precisely what NeRFs can do, but by using AI to fill in the missing images to create a 3D field of view! Here’s an example of NeRFs in action in a McDonald’s ad:
The entry sequence here was not generated using computer animation or camera manipulation but AI via NeRFs! Here’s another cool example of a walkthrough of someone’s home that was generated using NeRFs:
Again this “flythrough” was never filmed on camera. It was generated using NeRFs with AI.
4/ RunwayML’s Text-To-Video model:
The last advancement worth highlighting is RunwayML’s video synthesis model, with Gen-2 launching at the end of March:
RunwayML's video generation models, such as Gen-1, allow users to transform existing videos based on text, image, or video inputs. Functionally, it works similarly to a style transfer tool, but instead of applying filters, it generates entirely new videos as outputs. For example, users can upload a video and apply an aesthetic or theme to give it the look of a watercolor painting, charcoal sketch, or other visual styles. The output of the generative AI can often result in unusual and creative effects, such as warping between frames, changing limb sizes, or melting and smearing features.
Runway AI Gen-2 is a significant improvement over Gen-1. It introduces the capability to generate full videos solely based on text descriptions, whereas Gen-1 could only modify existing videos using AI-generated overlays. Gen-2 combines the best qualities of both generations by allowing users to apply the composition and style of an image or text prompt to the structure of a source video, resulting in the generation of entirely new videos.
Here’s an example of Gen-2 in action:
5/ Track Anything
Finally, Track-Anything uses Meta’s recent Segment Anything and applies it to video to segment objects in a video:
This model lets characters easily be tracked, segmented, and edited in scenes without needing a green screen!
AI and the future of video
Since these advancements in generative video have landed in the last two months, many creators have been combining them together with generative images in exciting ways.
One of my favorites is Marvel Masterchef, which combines MidJourney, RunwayML, and Audio Synthesis:
I loved this example in particular because its combination of existing IP from Marvel, as well as the likeness of it, ’s famous actors and a Gordon Ramsey deepfake, is an excellent example of what the future of AI content looks like. As I mentioned in my last post on AI-generated music, I believe that licensing existing content, characters, and brands is going to be a massive new opportunity in entertainment:
Artists, too, stand to benefit from this shift. They could license their music to these platforms and receive royalties when their likeness is used in AI-generated tracks. Mapping generative content back to an artist's likeness may be achieved by analyzing the AI-generated prompt or machine learning-based labeling of the track after its creation.
Similar to my predictions for how music consumption will change because of AI, I think the same will be true with video. Movies and TV shows will likely become more personalized, interactive, and dynamic as AI is used not just in the video production process but end-to-end in generating the video itself! We might also see video games and movies merge into a single experience thanks to technologies like NeRFs.
I’m excited to see where this all leads and will be keeping an eye on the space closely over the next few months!
Thanks for reading The Hitchhiker's Guide to AI! Subscribe for free to receive new posts and support my work.
Neural Radiance Fields (NeRF) is a pretty cool technique used in computer graphics and machine learning. In simple terms, it's a way to create realistic 3D images or scenes using artificial intelligence. Let's break it down a bit so it's easier to understand.
Imagine you're taking photos of an object from different angles. Each photo captures a part of the object's appearance, including color, texture, and lighting. Neural Radiance Fields use AI to combine these photos and create a detailed 3D model of the object.
Here's how NeRF works:
Input: You start by feeding the AI a bunch of 2D images of an object or scene taken from various angles and viewpoints.
Neural Network: The AI processes these images using a neural network (a fancy term for a computer program inspired by the human brain). The network tries to learn the important features and relationships between the images.
3D Representation: The AI creates a continuous 3D representation of the object or scene. It does this by predicting the color and opacity (how see-through something is) of each point in the 3D space.
Rendering: Using this 3D representation, the AI can render new images of the object or scene from any angle or viewpoint.
The magic of NeRF is that it can generate very realistic and high-quality 3D images even when the input images are not perfect or have missing information. This makes it a powerful tool in areas like virtual reality, video games, and movies, where realistic 3D graphics are essential. ↩